3 Presentations
Understanding slot machine math can be daunt ing for even the most seasoned individual. You do not hav e to be an accountant, analyst, or even a statistician to understand the fundamentals of slot math. The take-away is to know why this math is so important in monitoring performance and compliance at your property. Slot.It, an Italian slot car company founded in 1988, has revolutionized the slot car racing world establishing itself as the reference brand for upgrade parts and competition slot cars. The collection of Slot.it slot cars offers world-class features, including the Slot.it V12 motor, Slot.it gears and aluminum wheels.
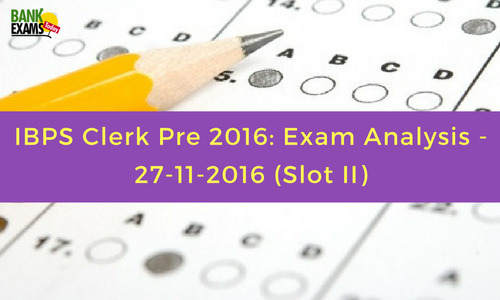
Robust Portfolio Optimization with Copulas, Creating a Portfolio Strategy for Periods of Crisis
Conditional value-at-risk (CVaR) is widely used in portfolio optimization as a measure of risk. CVaR is clearly dependent on the underlying probability distribution of the portfolio. We show how copulas can be introduced to any problem that involves distributions and how they can provide solutions for the modelling of the portfolio. We use this to provide the copula formulation of the CVaR of a portfolio. Given the critical dependence of CVaR on the underlying distribution, we use a robust framework to extend our approach to Worst Case CVaR (WCVaR). WCVaR is achieved through the use of rival copulas. These rival copulas have the advantage of exploiting a variety of dependence structures, symmetric and not. To investigate the advantage of such dependence structure we proceed with an empirical analysis. In our analysis we perform stock portfolio optimization using WCVaR and we compare the portfolio performance to a portfolio where the risk is modelled using Gaussian distribution. This analysis is applied to data from different stock markets around the world. Our empirical analysis shows that the WCVaR portfolio is more suitable to asses market risk in periods of crisis while the Gaussian portfolio is more suitable for tranquil periods.
Optimal Stopping for Portfolio Management
Optimal Stopping problems (OSP) offer a substantial improvement in terminating a procedure at an earlier stage thus in real-life problems saving costs of additional observations. The focus of this paper is on the application of an OSP to the area of Portfolio Management.
The challenging nature of portfolio management in today's fragile state of the markets has established that apart from protecting gains, limiting losses is crucial to portfolio managers. A simple example to illustrate its importance is if a loss of 50% occurs on a £100 investment then a subsequent profit of 100% is required just to break even. A number of practical stop loss tools are available including the Trailing Stop, the MACD and the Bollinger bands. However, all these tools do not allow future possible scenarios to be considered in the optimisation of the exit time.
A new Finite Horizon OSP for portfolio management, namely the Portfolio Management Problem (PMP), is introduced that allows future possible scenarios to be considered in the optimisation of the exit time. The PMP aims at maximizing the expected return of an investor investing in a portfolio. A Dynamic Programming approach is proposed to solve the PMP as it best fits the PMP objective; the optimal policy. The subsequent DP algorithm developed is capable to solve problems graphically represented with a state space tree of up to approximately 33.5 million vertices.
However, the applicability of the PMP is limited to problems that, on one hand, assume a market with no memory and, on the other hand, assume no constraints have been imposed by either the organisation where the fund belongs to, or by the fund manager. In view of adding some realism into the model, this paper addresses the latter requisite by introducing mean reversion, a stop loss and a target return into the PMP model.
A problem definition and formulation for the mean-reverting PMP with constraints is presented. An illustrative example is demonstrated to explain the proposed mathematical formulation and its computational implementation. The computational challenges, firstly, due to an exponentially growing state-space tree, and secondly, due to Mean Reversion requiring all paths in the state space tree to be available for interrogation, are discussed. Finally, an extensive computational study including results for different problem sizes, MR rules and constraints is presented.Venture Capitalist Portfolio Selection under Probabilistic and Possibilistic Risk
This paper views a venture capitalist's (VC's) portfolio selection problem under two types of uncertainties, i.e., probabilistic risk and possibilistic risk. A VC faces such a complex situation, when it has under analysis several potential target companies of which some are handled in probabilistic terms, while others call for a possibilistic treatment. A possibilistic treatment is suitable for cases, where uncertainty is very high. This can be due to lack of available statistical information, because investment targets are often privately owned small companies with limited public information, possibly totally without past sales, without market values, there don't exist comparable firms to allow comparables-based valuations, and their value largely depends on intangibles and their strategic future actions.
The total value of a VC investment is defined to consist of two parts (cf. Smit and Trigeorgis, 2007): (i) the base value of a target, which depends primarily on it’s operating assets and (ii) the value of strategic growth opportunities, which depends largely on the combined strategic capital of the target and the VC and the inherent flexibility of strategic future actions. We present a real options approach for such venture capitalist setup: standard NPV methods are applicable to value the base (i) and real options methods are applicable to value the growth opportunities (ii). In our approach some targets’ strategic parts are valued probabilistically using Datar-Mathews real options method (Datar and Mathews, 2004) and other targets’ strategic parts are valued possibilistically using the fuzzy real options method (Collan et al 2009). The applied methods are selected for their intuitivity and practicality; other real options methods are can be used accordingly.
The approach of the paper extends the fuzzy real options company valuation method of Kinnunen (2010) and Collan and Kinnunen (2011) to venture capitalist framework and allows both fuzzy and probabilistic components. The VC's portfolio selection problem (Georgescu and Kinnunen, 2012) corresponds to a situation in which some return rates on investments are described by random variables, while others by fuzzy numbers. This paper unifies Markowitz probabilistic model and a possibilistic portfolio selection model resulting in the optimal solution of the mixed portfolio problem faced by a VC. The paper discusses the added value and the usefulness of the two-component approach for a VC and presents a spreadsheet-compatible calculation example to demonstrate the practicality of the approach.
Keywords: portfolio optimization; venture capital; corporate acquisitions, risk theory; possibility theory; probability theory
ReferencesCollan M, Fullér R and Mézei J (2009). Fuzzy Pay-off Method for Real Option Valuation. Journal of Applied Mathematics and Decision Systems.
Collan M, Kinnunen J, (2011). A Procedure for the Rapid Pre-acquisition Screening of Target Companies Using the Pay-off Method for Real Option Valuation, Journal of Real Options and Strategy 4(1): 117-141.
Datar V and Mathews S (2004) European real options: An intuitive algorithm for the Black-Scholes formula. Journal of Applied Finance 14: 7-13
Georgescu I and Kinnunen J (2012a). A Mixed Portfolio Selection Problem. Advances in Intelligent and Soft - Computing Distributed Computing and Artificial Intelligence 151: 95-102
Kinnunen J (2010). Valuing M&A Synergies as (Fuzzy) Real Options, Proceedings (CD-ROM) of the 14th Annual International Conference on Real Options, Rome, Italy, June 16-19
Smit HTJ and Trigeorgis L (2006). Strategic planning: valuing and managing portfolios of real options. R&D Management 36(4): 403-419
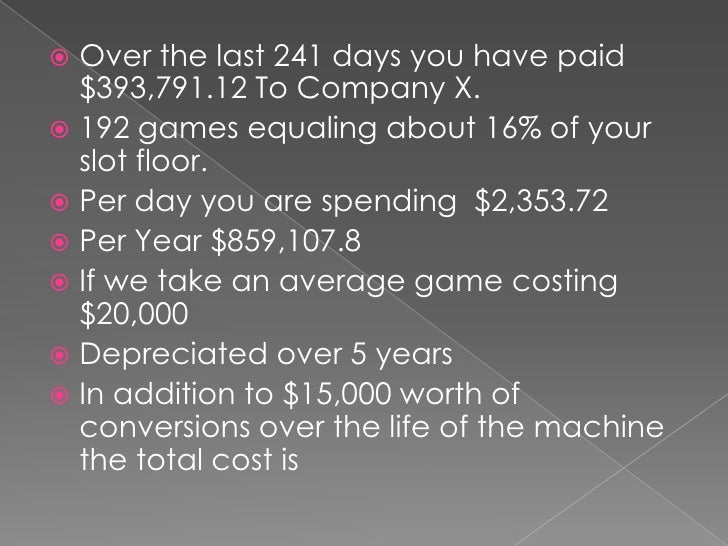
Slot 2 Analysis Cat 2019
Probably every single one of us has seen a slot machine at least once in our lives and besides the themes and colors they all look and sound the same, but are they? That is the question to ask!
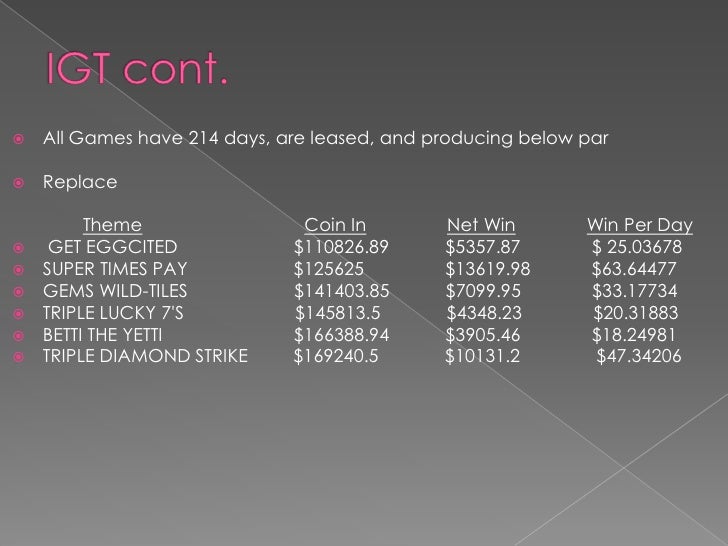
Slot machines are usually divided into two groups, Class II and Class III machines. It is more than just easy to distinguish them when you known this simple trick; just check out the corners of the electronic display and if you find a little bingo card there then you can be sure that you are playing a Class II slot machine. Now that you know how to guess what kind of slot you are playing on, let us move on to understanding what the difference between them actually is.
Slot Analysis Ii Notes
Who are you playing against?!
Now that is the biggest difference between Class II and Class III slot machines. Let us discuss Class II slots first; in this case you are playing against other players like you but the set of prizes are quite limited. This type of slots basically mimic the real game of Bingo. The game starts by hitting the spin button and you automatically join in the process. Certain number of slot machines are linked to the central processing computer that controls the game and calls out numbers and winner accordingly. One major difference between real-life Bingo and Class II slot machines is that you do not have to pay attention to the caller and search for the numbers on your card. At the end of the play, casino takes part of the income from the play and gives out the rest as cash prize to the winners.
You versus the Casino
This is the main principle of the Class III slot machines. You are simply playing against the casino. The slot machines are set up in a manner that it hold a certain part of the money played and redistributes the rest to the players. So, it is all about timing and luck. On the contrary to the Class II slot machines, Class III machines can make you a big winner.
To sum it up, Class II slot machines are played among some players simultaneously who battle for a single prize, while Class III machines can be played by lots of different people at different times and they all seek for the payout from a single machine.
Slot 2 Analysis 2019
Antoine Foxx
Slot Analysis Ii
33 year old male. Has visited Las Vegas several times as well as other great gambling places. Always having a good time. After having a little girl, he couldn’t travel as much so he started enjoying online gaming. Antoine is always looking for something different and interesting. Part time rock star but full time computer geek, he never seems to guarantee a balanced life. Enjoys Cajun food a lot!
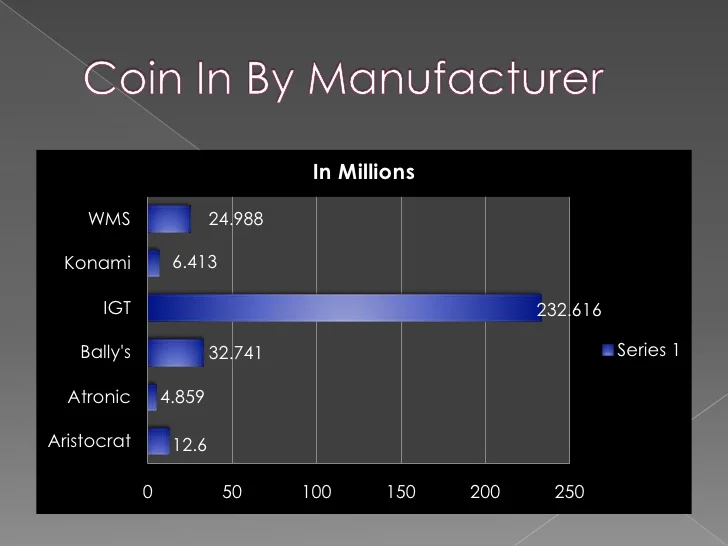